BaCAI Lectures
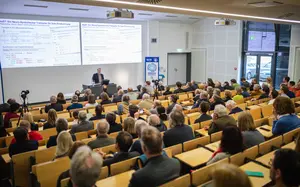
In the BaCAI Lectures, renowned speakers from various fields of artificial intelligence present their research. The target group is mainly university members due to the focus on research and the main language English, but the lectures are open to all interested parties.
Event details (unless otherwise stated):
- Location: WE5/00.022 (An der Weberei 5, Erba, Universität Bamberg)
- Start: 6 pm (c.t.)
- Duration: approx. 1 hour plus questions
- Language: English
Dates in the Winter term 2024/25
The dates will be announced before the start of the semester
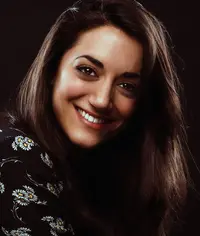
Title tba
About Ira Ktena, PhD
Ira Ktena is a Staff Research Scientist at Google DeepMindworking on Safe and Reliable Machine Learning research. Previously, she was a senior Machine Learning Researcher with the Cortex Applied Research team at Twitter UK, focusing on real-time personalisation while she carried out research at the intersection of recommender systems and algorithmic transparency. Their exploration on algorithmic amplification of political content on Twitter was featured by the Economist and the BBC, among others.
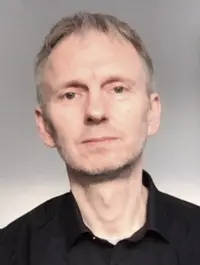
Uncertainty Quantification in Machine Learning: From Aleatoric to Epistemic
Due to the steadily increasing relevance of machine learning for practical applications, many of which are coming with safety requirements, the notion of uncertainty has received increasing attention in machine learning research in the recent past. This talk will address questions regarding the representation and adequate handling of (predictive) uncertainty in (supervised) machine learning. A particular focus will be put on the distinction between two important types of uncertainty, often referred to as aleatoric and epistemic, and how to quantify these uncertainties in terms of appropriate numerical measures. Roughly speaking, while aleatoric uncertainty is due to the randomness inherent in the data generating process, epistemic uncertainty is caused by the learner's ignorance of the true underlying model.
About Prof. Dr. Eyke Hüllermeier
Eyke Hüllermeier is a full professor at the Institute of Informatics at LMU Munich, Germany, where he holds the Chair of Artificial Intelligence and Machine Learning. He studied mathematics and business computing, received his PhD in Computer Science from Paderborn University in 1997, and a Habilitation degree in 2002. Before joining LMU, he held professorships at several other German universities (Dortmund, Magdeburg, Marburg, Paderborn) and spent two years as a Marie Curie fellow at the IRIT in Toulouse (France).
His research interests are centered around methods and theoretical foundations of artificial intelligence, with a particular focus on machine learning, preference modeling, and reasoning under uncertainty. He has published more than 400 articles on related topics in top-tier journals and major international conferences, and several of his contributions have been recognized with scientific awards. Professor Hüllermeier is Editor-in-Chief of Data Mining and Knowledge Discovery, a leading journal in the field of AI, and serves on the editorial boards of several other AI and machine learning journals. He is currently President of the European Association for Data Science (EuADS), a member of the Strategy Board of the Munich Center for Machine Learning (MCML), and a member of the Steering Committee of the Konrad Zuse School of Excellence in Reliable AI (relAI).
Past BaCAI lectures
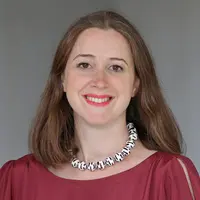
Why do LLMs need to worry?
Lecture in English
The Achilles heel of Natural Language Processing research has for a long time been the noise in its datasets, in particular inaccurate labels. While there has been a paradigm shift from small expert models trained on labelled datasets to large language models (LLMs) trained by largely unlabelled data, which are capable of solving a variety of tasks, the problems of overconfidence and bias persist. In this talk I will present some methods for estimating uncertainty in task-oriented dialogue and utilising it to automatically correct the labels in the underlying datasets. I will hypothesise how this may open the door to solving related issues in LLMs.
About Prof. Dr. Milica Gašić:
Milica Gašić is a Professor of the Dialog Systems and Machine Learning Group at the Heinrich Heine University Düsseldorf. Her research focuses on fundamental questions of human-computer dialogue modelling and lie in the intersection of Natural Language Processing and Machine Learning. Prior to her current position she was a Lecturer in Spoken Dialogue Systems at the Department of Engineering, University of Cambridge where she was leading the Dialogue Systems Group. Previously, she was a Research Associate and a Senior Research Associate in the same group and a Research Fellow at Murray Edwards College. She completed her PhD under the supervision of Professor Steve Young and the topic of her thesis was Statistical Dialogue Modelling for which she received an EPSRC PhD Plus Award. She holds an MPhil degree in Computer Speech, Text and Internet Technology from the University of Cambridge and Diploma (BSc. equivalent) in Mathematics and Computer Science from the University of Belgrade. She is a member of ACL, a member of ELLIS and a senior member of IEEE as well as a member of the International Scientific Advisory Board of DFKI.
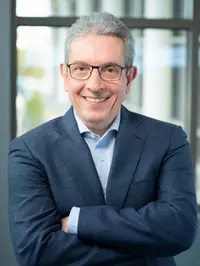
Probabilistic and Deep Learning Techniques for Robot Navigation and Automated Driving
For autonomous robots and automated driving, the capability to robustly perceive environments and execute their actions is the ultimate goal. The key challenge is that no sensors and actuators are perfect, which means that robots and cars need the ability to properly deal with the resulting uncertainty. In this presentation, I will introduce the probabilistic approach to robotics, which provides a rigorous statistical methodology to deal with state estimation problems. I will furthermore discuss how this approach can be combined using state-of-the-art technology from machine learning to deal with complex and changing real-world environments.
About Prof. Dr. Wolfram Burgard
Prof. Dr. Wolfram Burgard is a distinguished Professor of Robotics and Artificial Intelligence at the University of Technology Nuremberg where he also serves as Founding Chair of the Engineering Department. Previously, he held the position of Professor of Computer Science at the University of Freiburg from 1999 to 2021, where he established the renowned research lab for Autonomous Intelligent Systems. His expertise lies in artificial intelligence and mobile robots, focusing on the development of robust and adaptive techniques for state estimation and control. Wolfram Burgard's achievements include deploying the first interactive mobile tour-guide robot, Rhino, at the Deutsches Museum Bonn in 1997. He and his team also developed a groundbreaking approach that allowed a car to autonomously navigate through a complex parking garage and park itself in 2008. In 2012, he and his team developed the robot Obelix that autonomously navigated like a pedestrian from the campus of the Faculty of Engineering to the city center of Freiburg. Wolfram Burgard has published over 350 papers and articles in robotic and artificial intelligence conferences and journals. Additionally, he co-authored the two books “Principles of Robot Motion - Theory, Algorithms, and Implementations” and “Probabilistic Robotics”. In 2009, he was honored with the Gottfried Wilhelm Leibniz Prize, the most prestigious research award in Germany. He is a member of the Heidelberg Academy of Sciences and the German Academy of Sciences Leopoldina.
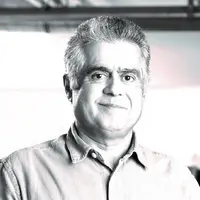
Particularity and Challenges of Machine Learning for Intra-operative Imaging
Towards precision and Intelligence in high intensity, dynamic environments
Lecture in English
Over the past decade, the rapid advancements in machine learning (ML) have revolutionized various fields, significantly impacting our lives. In this talk, we will delve into the realm of medical applications and explore the challenges and opportunities associated with integrating these cutting-edge technologies into computer-assisted interventions. Our primary focus will be on fostering acceptance and trust in machine learning and robotic solutions within the medical domain, which often necessitates the path through Intelligence Amplification (IA). Augmented Reality (AR) allows us to leverage IA to augment human intelligence and expertise, ultimately paving the way for the seamless integration of Artificial Intelligence (AI) and robotics into clinical solutions.
Drawing from some groundbreaking research conducted at the Chair of Computer-Aided Medical Procedures at both TU Munich and Johns Hopkins Universities, I will present a series of novel techniques developed to address the unique demands of medical applications. Specifically, we will explore their practical implementations in diverse areas, including Robotic Ultrasound Imaging, Multimodal Data Analysis, and Semantic Scene Graphs for Holistic Modeling of Surgical Domain. Furthermore, I will showcase compelling examples of how AR-solutions can serve as catalysts for embracing AI in computer-assisted surgery. By harnessing the power of IA, we can unlock the full potential of AI technologies, bolstering acceptance and driving the future of computer-assisted interventions. Join me on this enlightening journey as we navigate the intricate intersection of machine learning, medical advancements, and the path from intelligence amplification to artificial intelligence in healthcare.
About Prof. Dr. Nassir Navab
Prof. Dr. Nassir Navab is a full professor and director of the Laboratories for Computer Aided Medical Procedures (CAMP) at Technical University of Munich (TUM) and an adjunct Professor at Johns Hopkins University. He is also the director of biannual Medical Augmented Reality school series at Balgrist Hospital in Zurich. He is a Member of Academia Europaea and received the prestigious MICCAI Enduring Impact Award in 2021 and IEEE ISMAR 10 Years Lasting Impact Award in 2015. In 2001, while acting as distinguished member of technical staff at Siemens Corporate Research (SCR) in Princeton, he received the prestigious Siemens Inventor of the Year Award for the body of his work in interventional imaging. He also received the SMIT Technology Innovation Award in 2010. He is also honored as Medical AR Pioneer in AWE XR hall of fame in 2024. His students have received many paper awards including 15 awards at the prestigious MICCAI events, 5 at IPCAI, 2 at IPMI and 4 at IEEE ISMAR. He is Fellow of the MICCAI Society and acted on its board of directors from 2007 to 2012 and from 2014 to 2017. He is also an IEEE Fellow and Fellow of Asia-Pacific Artificial Intelligence Association (AAIA) and one of the founders of IEEE Symposium on Mixed and Augmented Reality (ISMAR) and has been serving on its Steering Committee since 2001. He is an Area Chair for ECCV 2024. He is the author of hundreds of peer reviewed scientific papers and over 100 granted US and international patents. As of April 2024, his papers have received over 76,600 citations and enjoy an h-index of 117.
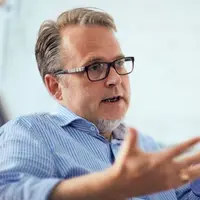
How to make artificial intelligence more human
The relationship between humans and machines, especially in the context of artificial intelligence (AI), is characterized by hopes, concerns and moral questions. On the one hand, advances in AI offer great hope: It promises solutions to complex problems, improved healthcare, more efficient workflows and much more. But at the same time, there are legitimate concerns about control over this technology, its potential impact on jobs and society, as well as ethical issues related to discrimination and the loss of human autonomy. The lecture will highlight and illustrate the complex tension between innovation and moral responsibility in AI research.
About Prof. Dr. Kristian Kersting
Prof. Dr. Kristian Kersting is co-director of the Hessian Center for Artificial Intelligence (hessian.AI) and heads the AI and Machine Learning department at TU Darmstadt. His research includes Deep Probabilistic Programming and Learning and Explainable AI. He is a Fellow of the Association for the Advancement of AI (AAAI), the European Association for AI (EurAI) and the European Lab for Learning and Intelligent Systems (ELLIS), book author ("Wie Maschinen lernen") and winner of the "Deutscher KI-Preis 2019". He writes a monthly AI column in the magazine "Welt".